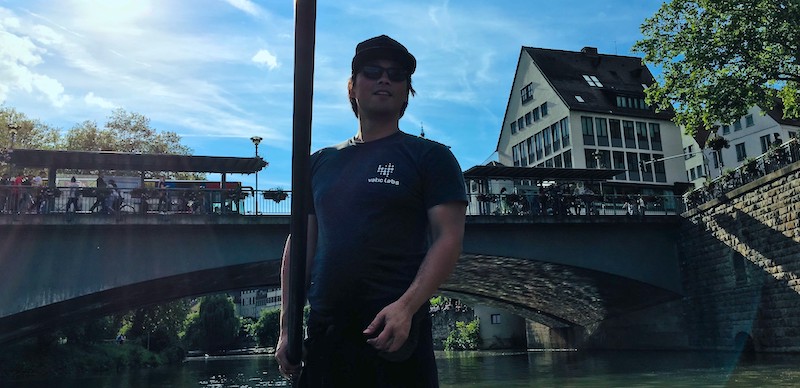
CV • Research Statement • Github • Google Scholar • DBLP • ResearchGate • ORCID
I have a broad interest in machine learning (ML). I am dedicated to understanding the underlying principles that enable autonomous agents to acquire knowledge effectively from their experiences and to utilize this understanding in designing novel machine learning (ML) algorithms capable of engaging in rational interactions with complex environments. Check out my publication and research pages for more information.
Welcome
Hallo! My name is Krikamol Muandet (ไกรกมล หมื่นเดช). I am currently a chief scientist and tenure-track faculty (fast track) at CISPA - Helmholtz Center for Information Security which is within the Helmholtz Association where I lead the Rational Intelligence (RI) Lab. From 2018 to 2022, I was a research group leader affiliated with the Empirical Inference Department at Max Planck Institute for Intelligent Systems, Tübingen, Germany. From January 2016 to December 2017, I was a lecturer at the Department of Mathematics, Faculty of Science, Mahidol University in Thailand. I graduated summa cum laude with a PhD degree specializing in kernel methods in machine learning. My PhD advisor was Prof. Bernhard Schölkopf. I also obtained a master’s degree with distinction in machine learning from University College London (UCL), United Kingdom. At UCL, I worked primarily in the Gatsby Unit with Prof. Yee Whye Teh.
My research interest lies in the area known as "machine learning". I am particularly interested in, for example, statistical learning theory, kernel methods, causal inference, counterfactual prediction, and algorithmic game theory. When I am not doing research, I enjoy reading books (on topics related to philosophy, psychology, history of science, etc) and watching movies. I also like outdoor sports such as bouldering, climbing, and snowboarding.
I am indebted to all my students and collaborators for our exciting collaborations. I always seek new collaboration, so I will be glad to hear from you.